As I write this, high-level US officials are still discussing the debt ceiling. That’s the maximum amount of money that the US federal government can officially owe. If the debt ceiling isn’t raised, then the US will end up defaulting on its loans — largely, but not only, meaning that it won’t make its regular payments to bondholders.
That would be rather bad, both for the US economy and for the world as a whole. That’s because US treasury bonds have long been seen as the most stable form of investment out there. You won’t make tons of money with treasury bonds, but you can be sure that you’ll get your money back. Which is why companies, governments, and individuals all buy treasury bonds — you might not earn that much interest, but at least you’ll get more than you would in a bank savings account.
If the US doesn’t pay its debts, then that raises all sorts of questions and causes lots of problems. Which is why financial markets are all talking about why the US needs to raise its debt ceiling in the coming days, before any of that would
This week, I thought we could look at data having to do with US debts. But I also thought that it would be fun and interesting not to write the Pandas code ourselves, but rather to ask ChatGPT to do it for us. Today, I’ll walk you through how I solved this problem, what’s going on behind the scenes, and even what obstacles I encountered in achieving my solution. I’m also going to record a video (which I’ll put on YouTube) walking through this solution, if you prefer to see it happen on screen.
Setup and configuration
In just six months, ChatGPT has become one of the most popular and best known technology products ever. I’ve read that over 100 million people signed up to use it within the first few weeks of it being released, which is pretty astonishing. (Even more so, if you consider that OpenAI hasn’t advertised its product at all; everything has come via word of mouth, social media, and the press.)
From the start, people noticed that ChatGPT was able to write code, along with poetry, songs, and short screenplays. (It can also read code and diagnose a variety of problems in it, which is pretty amazing.) But that was all with the first model, known as GPT-3.5. A newer model, GPT-4.0, is more sophisticated in a variety of ways — but it’s only open to paid subscribers, currently at a price of $20/month.
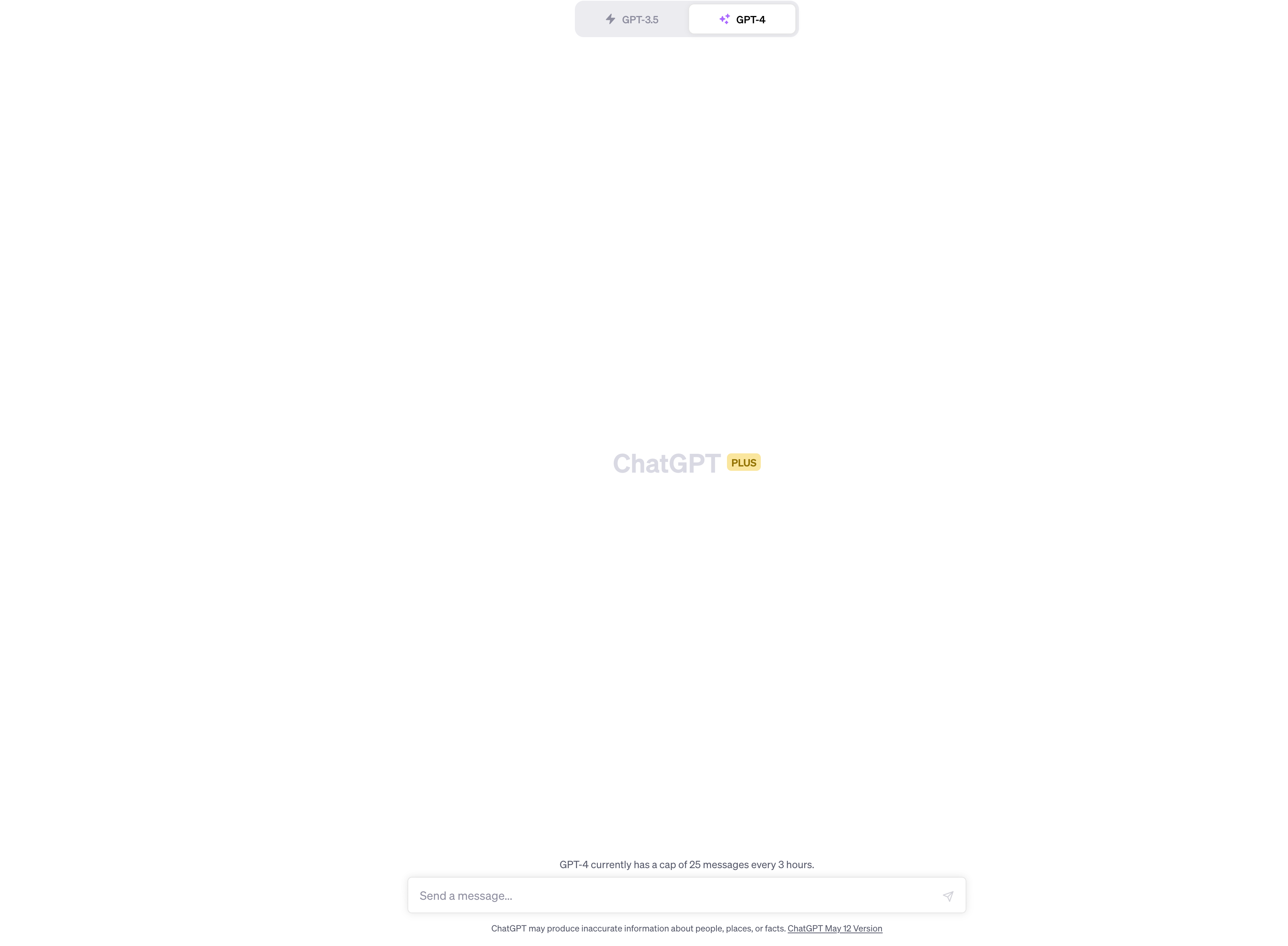
The above screenshot shows what it looks like when you are subscribed to ChatGPT Plus. At the top of the screen, you can select between the GPT-3.5 model, which runs faster and has no limits, and the GPT-4.0 model, which runs slower, is limited to 25 queries every three hours, and is more sophisticated.
If you’re subscribed to ChatGPT Plus, and if you’re using the GPT-4.0 model, then you can also choose from one of several options:
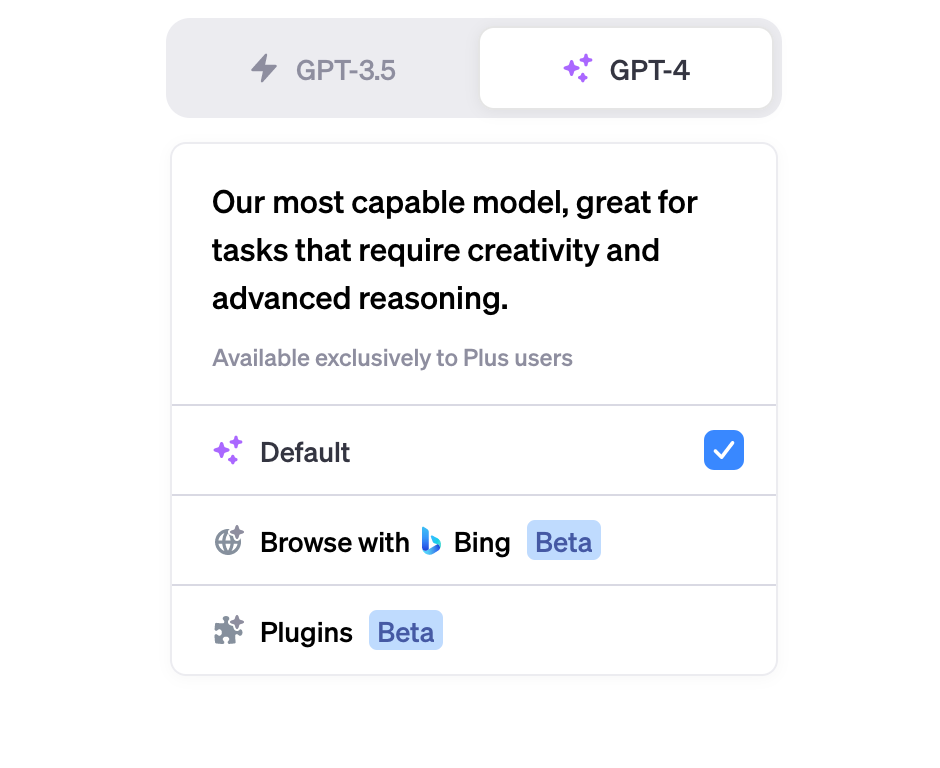
The above menu, visible when your mouse cursor hovers over the GPT-4 name, lets you optionally select to include browsing with Bing (i.e., including up-to-date Internet searches in your chat responses) or to use plugins. For our purposes, I’m going to select plugins:
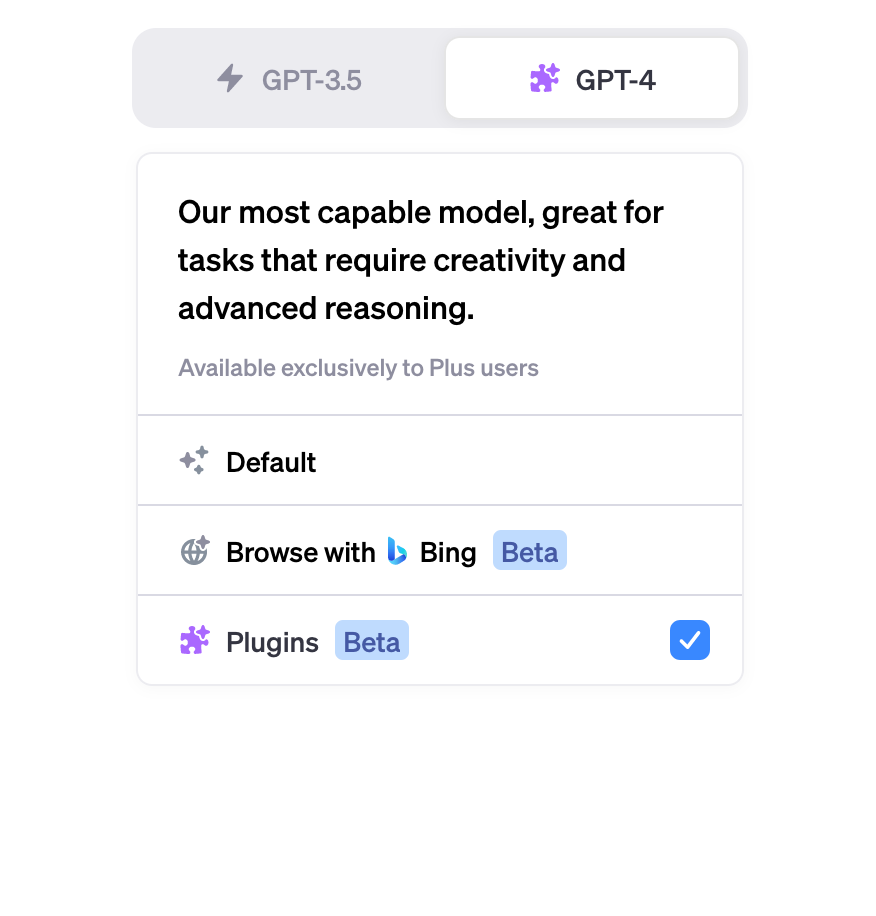
Once you select “plugins,” you will then see a menu at the top of the screen where you can select which plugins you want to install.

My menu shows three plugin icons, the ones that I have activated. If you haven’t selected any, you’ll have to pull down the menu. That’ll show you the list of installed plugins, including those that you have activated. It’ll also let you go to the plugin store.
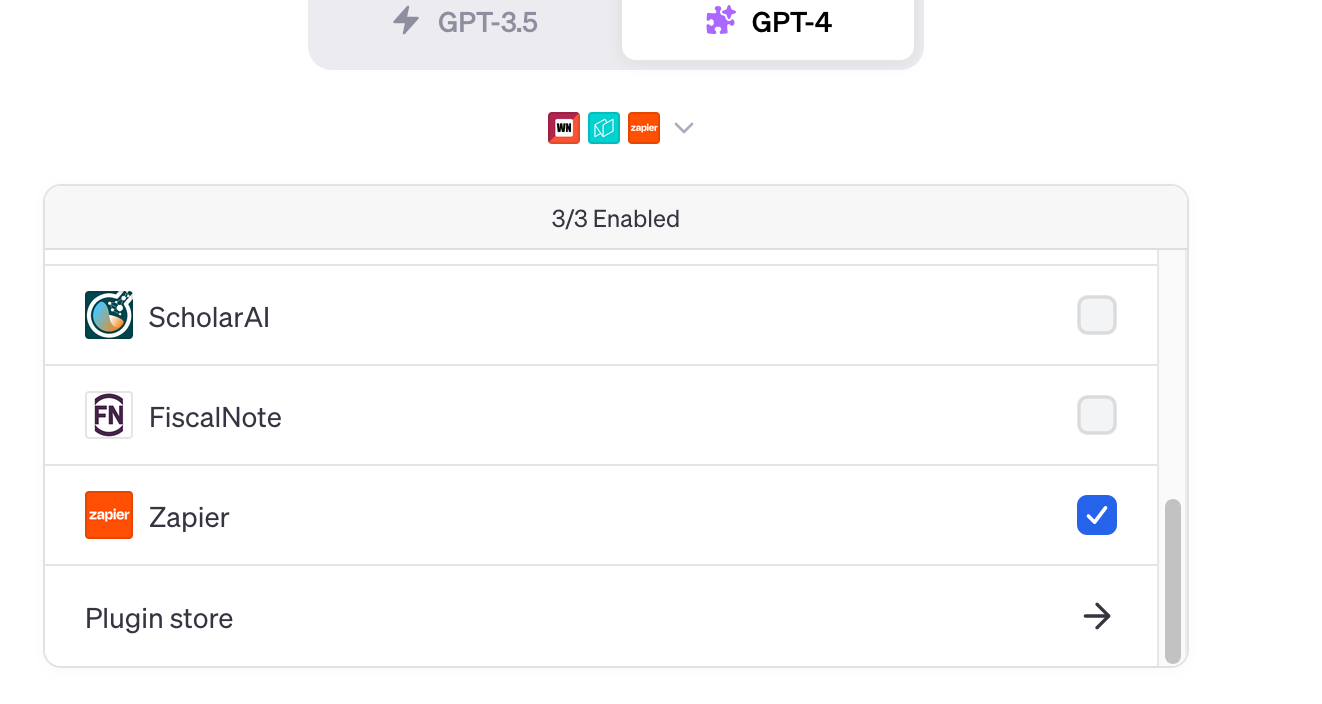
Clicking on the “plugin store” link brings you to this page:
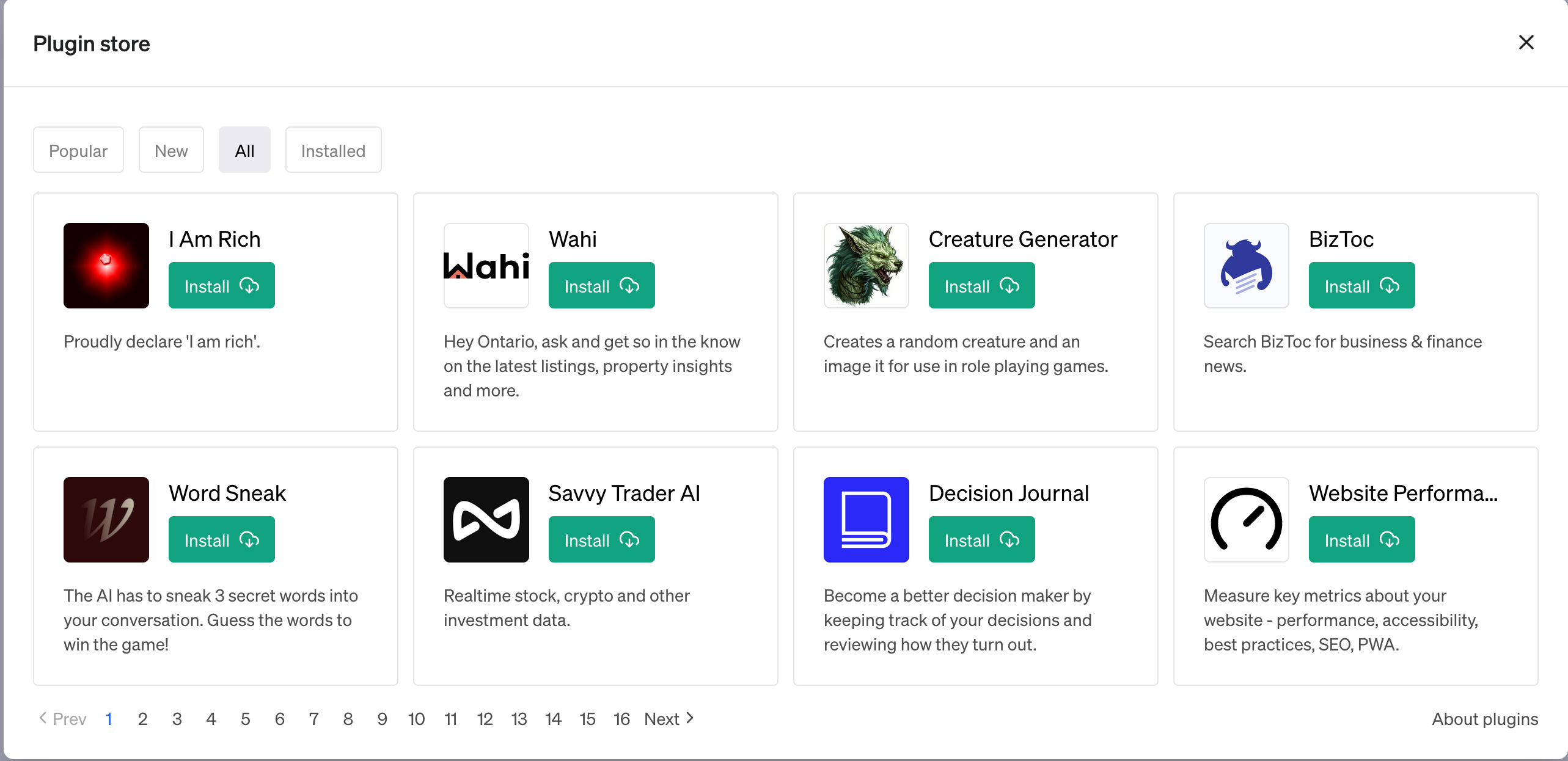
For today’s exercise, we’ll be using Noteable. Find the plugin, click on “install,” and if you haven’t yet installed other plugins, it’ll be activated as well (with a blue checkmark). In the days since I started to use ChatGPT Plus and the plugin store, the number of available plugins has increased dramatically.
If you don’t see the Noteable plugin, it might be because they’re updating the software. Every update apparently requires OpenAI to approve the new version, and people who viewed my YouTube video from a few days ago complained that it had disappeared from the plugin store, before reappearing a little while later. So if you don’t see it, just wait a bit.
In order to use the Noteable plugin, you’ll need to create a free Noteable account. Indeed, from speaking with someone at Noteable earlier this week, I learned that you can create as many projects and notebooks as you want on the free version of the app. The only limitations are that you’re on a small instance of Amazon’s EC2 virtual machines, which might not have the memory or CPU to handle larger data analysis tasks. Also, if you don’t use the Noteable system for some time — I can’t remember precisely how long — then the machine shuts down. Your notebooks will still be available, but they’ll need to be rerun to keep their state. You can pay for an enterprise account, at $120/person, which gives you a private machine and more options for EC2 instances — but it sounds like the free version of Noteable will be more than adequate for my needs at the moment.
After you’ve created a Noteable account, you’ll need to create a project, which you can think of as a directory.
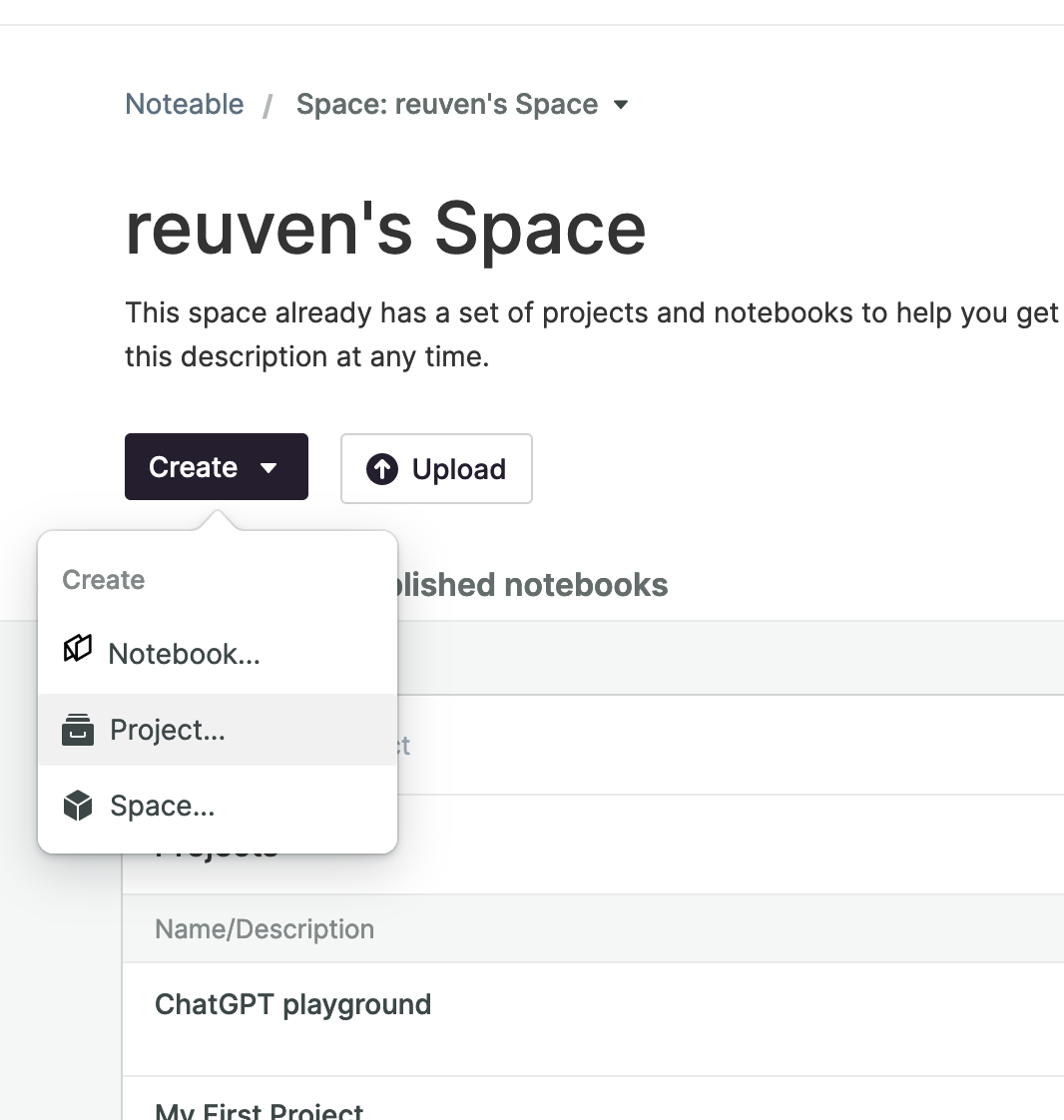
The above is what it looks like when I want to create a new project in my default Noteable space. Below the “create” menu, you can see that I already have a project called “ChatGPT playground,” which is where I’ve been doing my experimenting.
Now that Noteable is set up, I can go back to ChatGPT plus, making sure that I’m using GPT-4 and that the Noteable plugin is set up correctly. (There’s an authentication step that I’m forgetting or missing, but I remember it being very straightforward. I also remember that ChatGPT was very good at telling me what steps remained in order to finish setting up Noteable.)
Before continuing, we’ll also need to tell ChatGPT which Noteable project it should connect to via the plugin. You can do this by grabbing the full URL of your Noteable project, and then saying to ChatGPT:
Please use the Noteable project [INSERT_URL_HERE] for all notebooks.
Now that we have ChatGPT Plus, the Noteable plugin, and Noteable all set up, we can ask ChatGPT to perform all sorts of tasks for us. If the task has to do with downloading or analyzing data, then it’ll ask Noteable to do it.
This is a big shift: Instead of ChatGPT producing the Python and Pandas code that we could copy into our own Jupyter notebooks and run, here the AI is producing the code and then running it in a notebook. And the code that it produces, while not perfect, is still pretty darned amazing.
I’ve long thought that programming languages are frustratingly specific and pedantic because that’s the only way to avoid the ambiguity of natural language. Describing a technical task in English sounds like a great idea, but you can quickly discover many limits and gray areas. So when I posed yesterday’s question about the federal debt, it was after many, many hours of playing with ChatGPT, writing, editing, and repeating my questions in different ways to get the answer that I wanted. For me, it was frustrating to see how ChatGPT was so smart at turning my English into code — and yet so dumb at failing to understand what I was talking about.
Answering our questions
Yesterday, I posed 10 questions about the debt ceiling, and asked you to use ChatGPT to answer them. As you might have guessed, there wasn’t much work to do — because the questions, as I posed them, were precisely what I gave to ChatGPT. And I got the answers I was looking for. It was pretty amazing.
That’s not to say that things went swimmingly well all of the time. I sometimes ran up against ChatGPT’s limit of 25 queries every 3 hours. I sometimes saw ChatGPT get it wrong, realize that something was wrong, back up, and then try again. Sometimes, this retry worked perfectly, other times it didn’t, and at still other times it seemed to get stuck in an infinite loop. I spent a lot of time figuring out how to pose these questions, especially the questions at the end about which countries own American debt.
By the way, a nice thing about the GPT-4 model is that it accepts very long queries. So even if you’re limited to 25 questions every three hours, you can stick a lot of text in a single query. I tried to put all of my questions into a single query, but that nearly always ended up backfiring on me.
Let’s go through the questions (which are also the answers!) one by one, and I’ll comment on what I did and what I saw.
Because the questions are also the ChatGPT queries that I executed, I’ll put them in code-style blocks, as opposed to numbered questions (which is my usual style here):
Create a Jupyter notebook. In that notebook, create one Pandas data frame with the total amount of federal debt at https://fred.stlouisfed.org/series/GFDEBTN.
Use Seaborn to plot the total federal debt over the last three decades. The x axis should represent time, and the y axis should represent the amount of debt. Analyze the plot in Markdown, just below the plot.
When you ask ChatGPT to use a plugin, it shows you a little icon for each task it’s using the plugin for. When the Noteable plugin is in active communication with ChatGPT, it’s colorized, and says, “Using Noteable.”
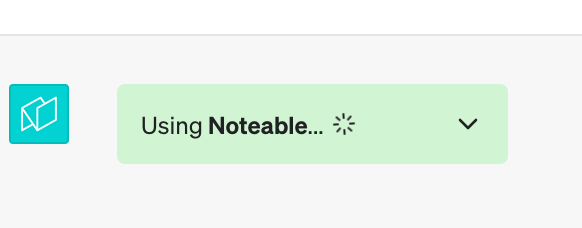
When it’s done, the icon changes to gray and says (in the past tense) “Used Noteable.”
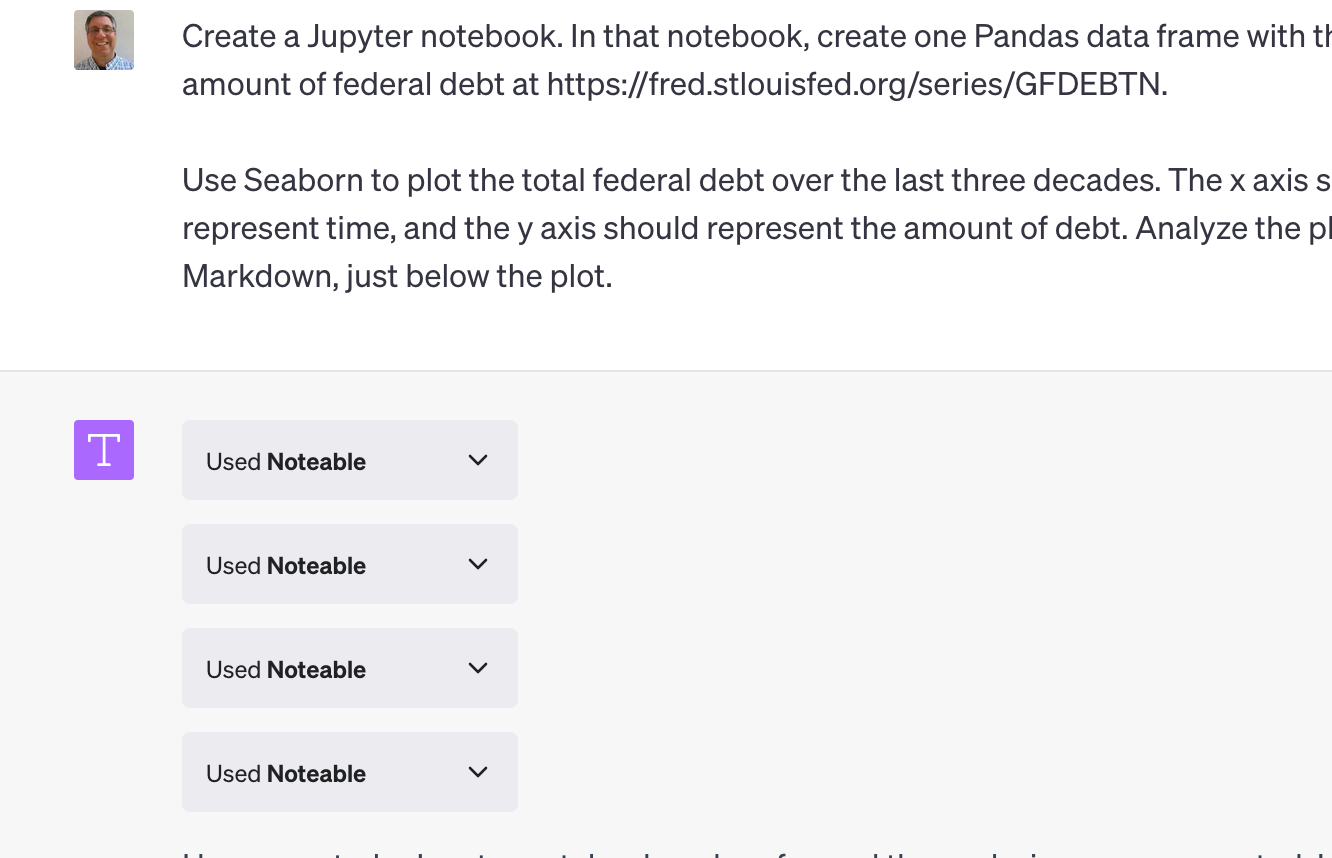
Notice that these icons have little arrows on them. You can open them up to see the JSON communication that went between the systems. In this case, ChatGPT communicated with Noteable in four ways:
- It created a notebook called “Federal debt analysis.” Not a bad name!
- It imported Pandas, Matplotlib, Seaborn, and datetime. It downloaded the data, asked for all date-looking columns to be parsed as dates (parse_dates=True), made the date column into the index, calculated today’s date, and then removed any rows from earlier than 30 years ago.
- It created a Seaborn line plot, including an appropriate title.
- It analyzed the plot, and gave an explanation in Markdown following the plot. The analysis wasn’t super insightful, but it wasn’t bad.
Pretty impressive all around, I have to say. I tend to shy away from raw Matplotlib calls, preferring to use Seaborn’s own API. So I probably would have called methods on “plt” much less. But… it got the job done, right?
Here was the plot it produced:
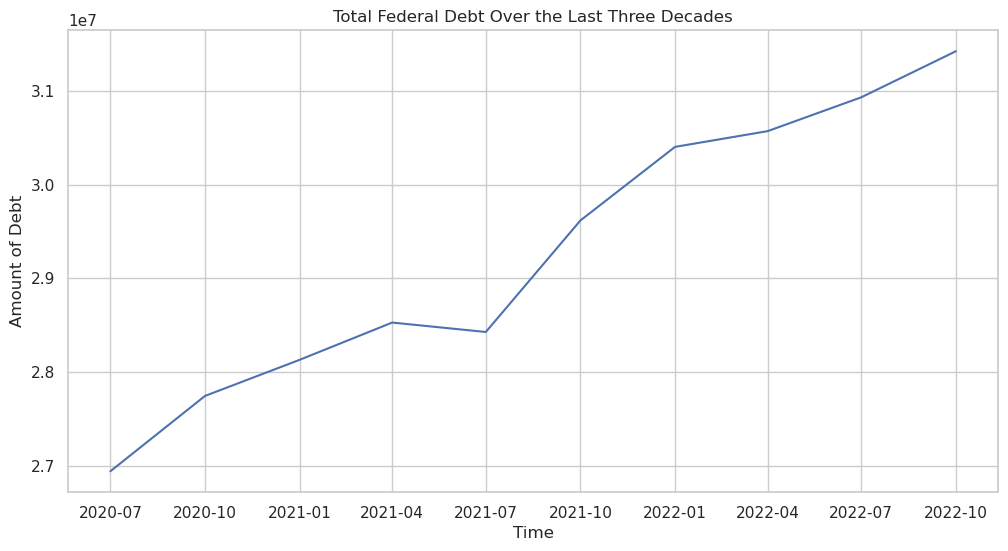
In the same notebook, download https://fred.stlouisfed.org/series/A091RC1Q027SBEA (interest payments on the Federal debt) into a separate data frame.
Use Seaborn to plot interest payments over the last three decades. The x axis should represent time, and the y axis should represent the interest payments. Analyze the plot in Markdown, just below the plot.
I learned the hard way that if you don’t tell ChatGPT to use the same Jupyter notebook, you can accidentally end up creating a new one. So I was explicit about using the same notebook, but also specifying a different data frame.
Other than that, the code was pretty similar this time around. It was smart enough to use a different variable name (df_interest). It was also smart enough to incorporate its previous analysis in this one, comparing the total federal debt with interest payments on that debt.
Here was the plot it created:
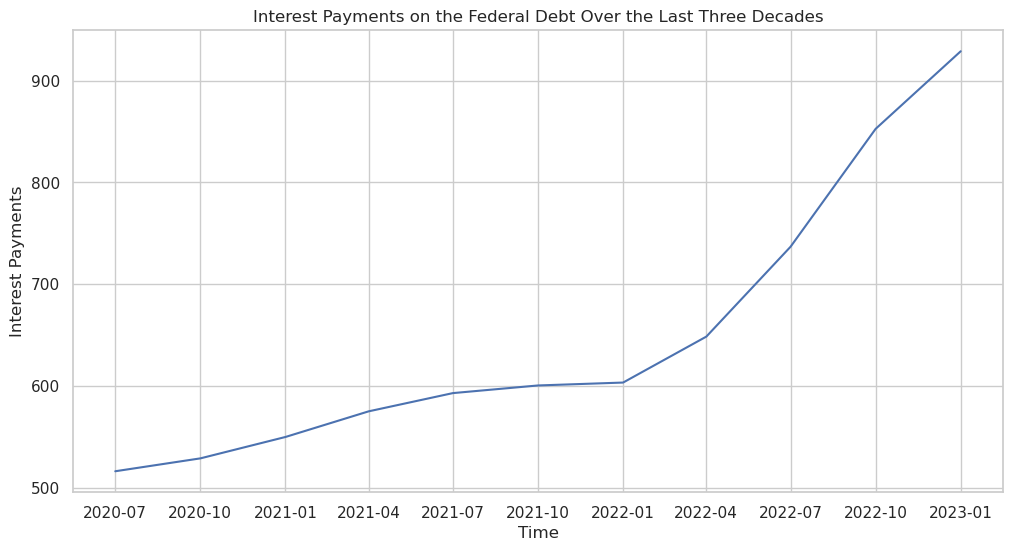